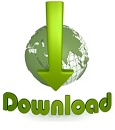
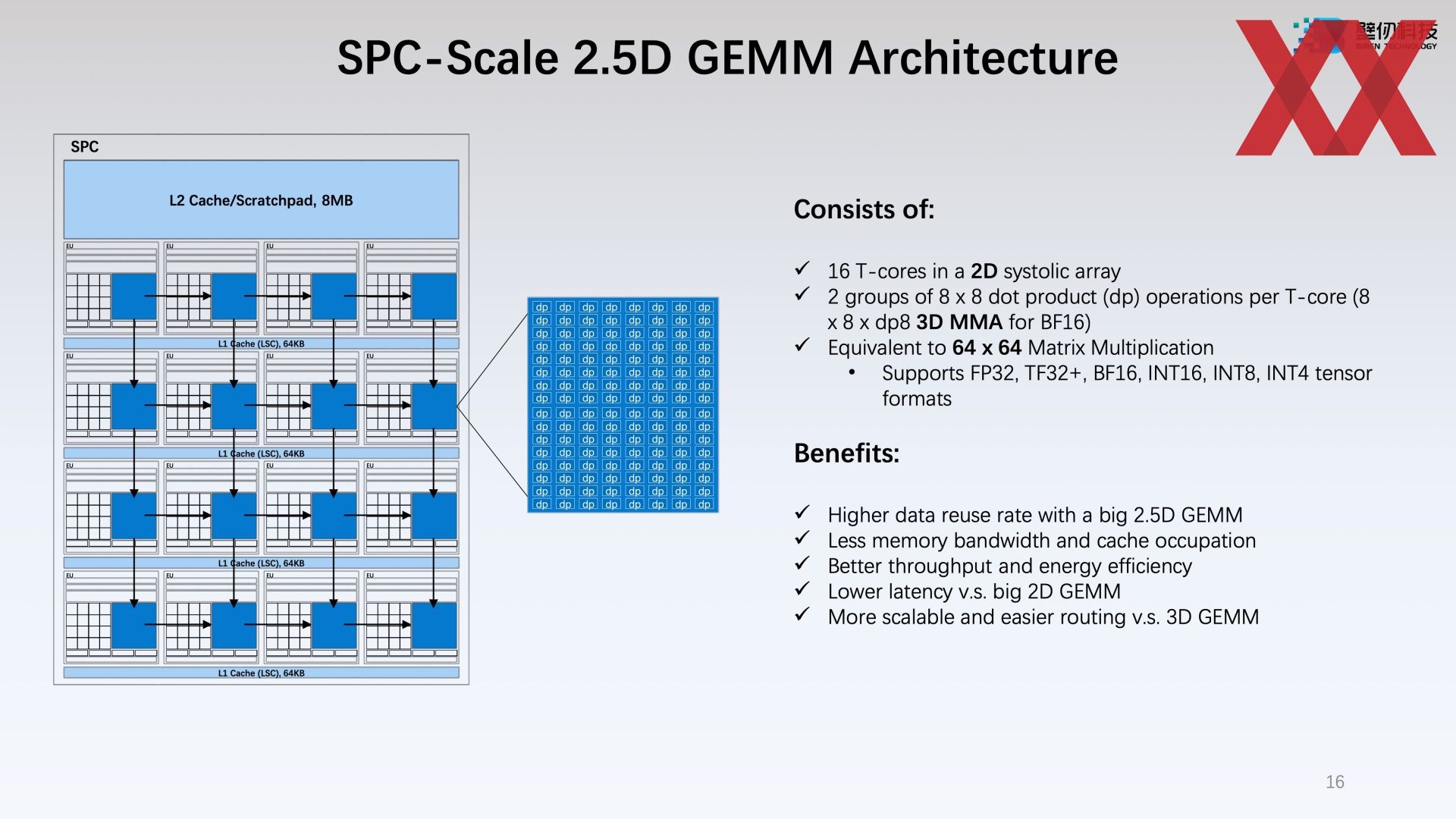
Compared to one T4, the speedup is 3.1x with two T4, and 10.4x with four T4. With the same number of GPUs, each model almost takes the same number of epochs to converge for T4 and V100-PCIe.įor GNMT model, the super-linear speedup was observed when more T4 GPUs were used. For Mask-R-CNN, V100-PCIe is 2.2x – 2.7x faster than T4. For SSD, V100-PCI is 3.3x – 3.4x faster than T4. For ResNet-50 v1.5, V100-PCIe is 3.6x faster than T4. The ResNet-50 v1.5, SSD and Mask-R-CNN models scale well with increasing number of GPUs. The following conclusions can be made based on these results: The training time in minutes was recorded for each benchmark. For each benchmark, the end-to-end model training was performed to reach the target model accuracy defined by MLPerf committee.
#Fp16 vs fp64 software#
Table 3: The hardware configuration and software detailsįigure 1 shows the performance results of MLPerf on T4 and V100-PCIe on PowerEdge R740 server. The T4 performance with MLPerf benchmarks will be compared to V100-PCIe. Table 3 lists the hardware and software used for the evaluation. All benchmarks were run on bare-metal without a container. The ResNet-50 TensorFlow implementation from Google’s submission was used, and all other models’ implementations from NVIDIA’s submission were used. The summary of MLPerf benchmarks used for this evaluation is shown in Table 2. The initial released version is v0.5 and it covers model implementations in different machine learning domains including image classification, object detection and segmentation, machine translation and reinforcement learning. MLPerf is a benchmarking tool that was assembled by a diverse group from academia and industry including Google, Baidu, Intel, AMD, Harvard, and Stanford etc., to measure the speed and performance of machine learning software and hardware. MLPerf was chosen to evaluate the performance of T4 in deep learning training. The specification differences of T4 and V100-PCIe GPU are listed in Table 1. T4 is the GPU that uses NVIDIA’s latest Turing architecture. The system features Intel Skylake processors, up to 24 DIMMs, and up to 3 double width V100-PCIe or 4 single width T4 GPUs in x16 PCIe 3.0 slots. The Dell EMC PowerEdge R740 is a 2-socket, 2U rack server. MLPerf performance on T4 will also be compared to V100-PCIe on the same server with the same software. This blog will quantify the deep learning training performance of T4 GPUs on Dell EMC PowerEdge R740 server with MLPerf benchmark suite. It was designed for High-Performance Computing (HPC), deep learning training and inference, machine learning, data analytics, and graphics. Turing architecture is NVIDIA’s latest GPU architecture after Volta architecture and the new T4 is based on Turing architecture.
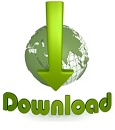